Listen to this article
|
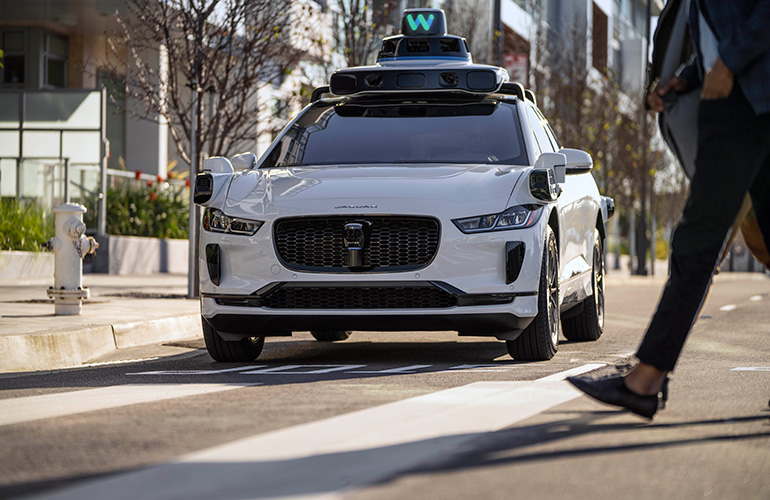
The VERSES team presented its initial experiments by illustrating its capabilities using the Waymo open dataset. | Source: Waymo
Autonomous vehicles need to be able to anticipate and react to people entering streets. VERSES AI Inc. today announced the initial results of its Genius Beta Partner collaboration on that topic. The cognitive computing company published a paper co-authored by research teams at Volvo Cars and VERSES.
The paper explained the use of algorithms from VERSES to predict the appearance of pedestrians, cyclists, and cars that are obscured behind stationary vehicles and objects. The companies claimed that the paper represents an advancement beyond the current capabilities of autonomous vehicles and artificial intelligence.
“As the automotive industry progresses towards fully autonomous self-driving cars, predicting where unseen obstacles like people or bicyclists may be or which trajectory they may be on has been a significant unsolved safety challenge,” said Gabriel René, CEO of VERSES.
“We believe the inability of current autonomous driving systems to overcome this hurdle is holding back the AV industry worldwide,” he added. “Volvo Cars is globally recognized for its unwavering commitment to vehicle safety. So, they were the perfect partner to work with to showcase how VERSES can help solve this problem.”
VERSES addresses driving uncertainty
The paper, titled “Navigation under uncertainty: trajectory prediction and occlusion reasoning with switching dynamical systems,” explores a way to help vehicles avoid people if they enter a street unexpectedly. It presents completed experiments illustrating capabilities using the Waymo open dataset.
The results demonstrate significant improvements in predicting animals, people, and objects entering the street, according to VERSES AI and Volvo.
VERSES said it is designing cognitive computing systems around first principles found in physics and biology. The Los Angeles-based company asserted that its flagship product, Genius, is a toolkit for developers to generate intelligent software agents that enhance existing applications with the ability to reason, plan, and learn.
How does the framework operate?
Predicting the future trajectories of nearby objects, especially under occlusion, is a crucial task in autonomous driving and safe robot navigation. The researchers said that prior works typically neglected to maintain uncertainty about occluded objects. Instead, they only predicted trajectories of observed objects through high-capacity models such as transformers trained on large datasets.
While these approaches are effective in standard scenarios, they can struggle to generalize to the long-tail, safety-critical scenarios, according to VERSES. This is why it set out to explore a conceptual framework unifying trajectory prediction and occlusion reasoning under the same class of structured probabilistic generative model, namely, switching dynamical systems.
The teams aimed to combine the reasoning of object trajectories and occlusions in a single framework. They did this with a class of structured probabilistic models called switching dynamical systems, which divides the modeling of complex continuous dynamics into a finite set/mixture of simple dynamics arbitrated by switching variables.
The main attractiveness of this class of models is that it provides a unified representation where hierarchical compositions generalize to both prototype-based trajectory prediction and object-centric occlusion reasoning, said the paper. For trajectory prediction, the switching variable represents the intent or behavior primitive chosen by the modeled object, where the execution of the selected intent generates trajectories prescribed by the attractor of the local dynamics.
For occlusion reasoning, the switching variable represents objects’ existence, which in turn modulates the prediction of their sensory measurements in combination with the scene geometry,” said René. “A potential advantage of this unified yet structured framework is that it could use efficient inference and learning algorithms while still being amenable to manual specification of specific critical components, such as scene geometry.”
Team uses Waymo data set to predict movements
To demonstrate the feasibility of this framework, the VERSES and Volvo team evaluated a minimal implementation on the Waymo open motion dataset to predict the motion of vehicles and pedestrians in occluded traffic scenes.
For trajectory prediction, the team compared the model’s prediction accuracy and uncertainty calibration against a few ablations. For occlusion reasoning, it visualized the model’s projections of potentially occluded pedestrian positions to show how uncertainty is maintained over time.
The team showed that both tasks can be embedded in the same framework and yet still be solvable with divide-and-conquer approaches. Its experimental results showed that, when conditioned on the same information, the closed-loop rSLDS models achieved higher predictive accuracy and uncertainty calibration.
“We believe this research project with Volvo Cars, part of our Genius Beta project, demonstrates a major advancement in autonomous vehicle safety capability. We expect the research project to pave the way for safer streets for pedestrians, cyclists, cars, robots, and beyond.”
VERSES said it plans to incorporate auxiliary information such as road graphs to improve prediction accuracy and to implement efficient inference algorithms that are particularly suitable to this family of models.
Tell Us What You Think!